Part 2 of Scaling AI - The AI revolution: redefining business models and investment strategies
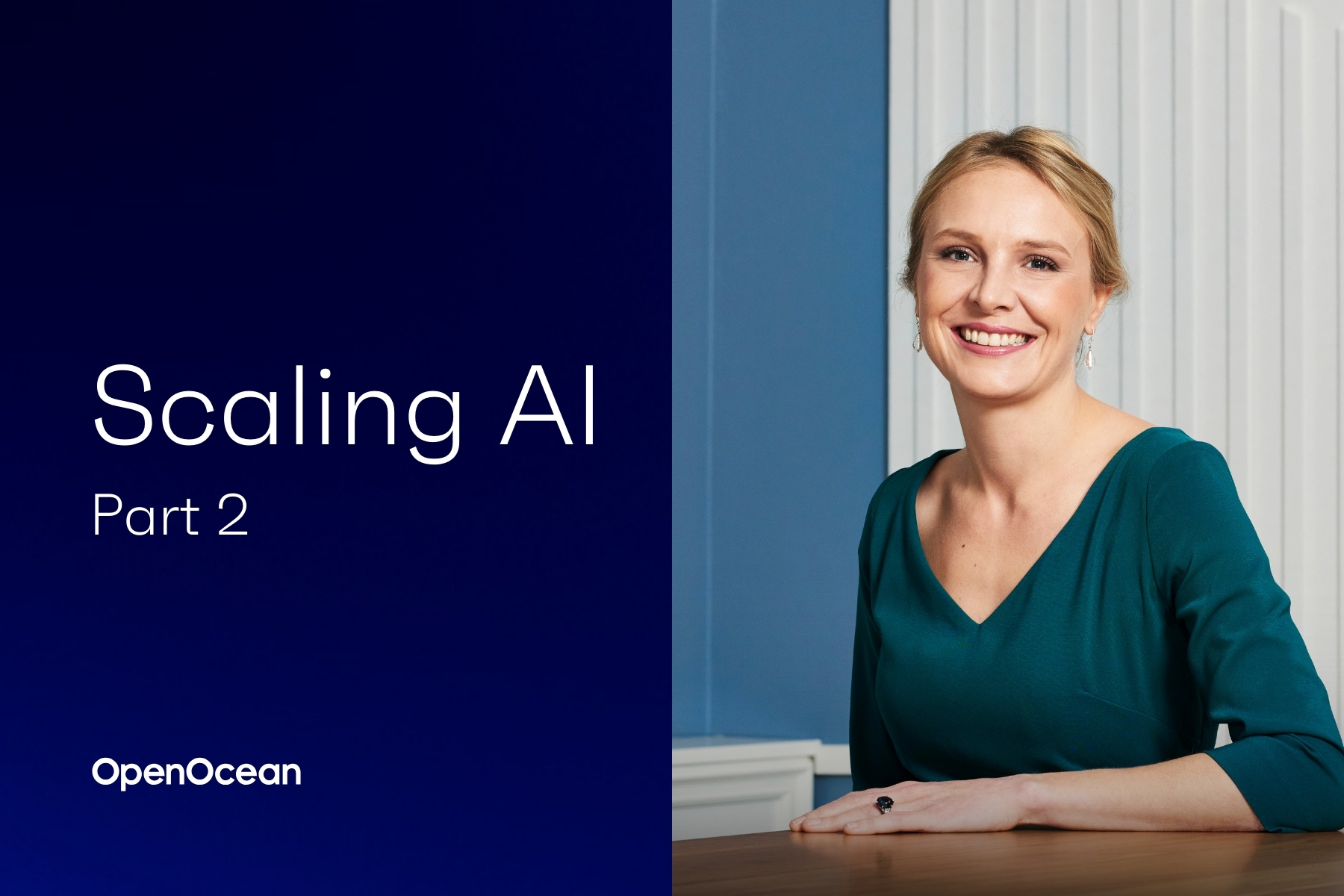
This is the second part of Scaling AI, a four-part series exploring how AI is transitioning from experimentation to delivering real-world impact for enterprises. As 2025 starts to unfold, the AI revolution enters a crucial stage – scaling.
After a decade of experimentation, we are now witnessing the widespread operationalisation of AI across industries. This shift is not merely a technological upgrade; it’s a fundamental transformation in how businesses operate, create and deliver value. AI is redefining business models, and understanding this new landscape is key for enterprises and investors alike.
New business models: where the value of AI is being created
As free open-source LLMs have closed the performance gap with closed models, as seen in benchmarks like the Arena ELO Index and DeepSeek R1’s recent performance, it is already becoming clear that monetising AI will become a challenge. According to Ibbaka’s report on AI monetisation, only 15% of B2B SaaS companies have successfully capitalised on AI so far, and we expect this to continue being difficult.
When the larger AI models widen their scope, smaller entrants face increasing challenges competing against the expanding offerings of the well-funded major players such as OpenAI and Anthropic. Data, once described as the "new oil," may now become the last defensible moat for AI companies. As algorithms become easier to access, proprietary high-quality datasets, such as unique customer behaviour data or diagnosed medical scans, will be a differentiator that defines market leaders.
Hence three critical areas are emerging for capturing long-term AI value:
- Data-related software
- AI infrastructure
- AI applications built on unique datasets
- Data-related software: the spades of the last defensible moat
The growing need for businesses to create custom AI models – whether driven by unique requirements or proprietary concerns – has placed data management at the centre of competitive strategy. Since 80% of corporate data is mainly unstructured, businesses have a real need to make their data AI-ready. Those that succeed will gain a major competitive advantage. This drives demand for software solutions that create data for AI use, creating opportunities for new data-related software to help businesses thrive in an AI-first world.
- AI infrastructure: the backbone of the revolution
AI infrastructure is a nascent industry but fast becoming the cornerstone of the AI economy. Many businesses are shifting towards AI-as-a-Service (AIaaS) models, allowing them to scale AI capabilities without the need for in-house infrastructure. While hyperscalers have already emerged, many segments of AI infrastructure still lack clear market leaders, as industry conventions have not been set yet. This area is an untapped investment opportunity, as scalable, model-agnostic infrastructure solutions will be the unsung heroes of the AI revolution.
- AI applications with unique datasets: the future of adoption
Automation use cases remain among the highest value creators, which leads to the expected rise in specialised AI models. These applications, built on unique, use-case-specific datasets, will be uniquely positioned to offer the accuracy, explainability and trust required for real-world automation. This is where AI adoption will accelerate, as businesses demand reliable solutions that integrate seamlessly and perform consistently in real-world situations.
The changing dynamics of business models and operations
Over the next 5–10 years, AI will reshape both operational strategies and business models across industries.
- A new variable cost: AI inference costs
One key distinction between AI and traditional software is the ongoing cost of running AI models. These relatively high variable costs make traditional business models – like subscription-based pricing – unsustainable for many AI-driven companies. As a result, usage-based or outcome-based pricing models are emerging as the primary way for businesses to scale profitably.
- Generative AI rises, but discriminate AI still reigns
While generative AI garners most of the media attention, discriminative AI models – focused on classification and decision-making – continue to create the most economic value. According to McKinsey, up to two-thirds of AI’s value will come from these non-generative applications, making it the primary driver of AI adoption.
- A shift toward smaller, focused models
Despite the ongoing pursuit of artificial general intelligence (AGI), large AI models are showing diminishing returns and potentially the need for a paradigm shift. In addition, the need for compute efficiency, explainability requirements and AI on the Edge trends, e.g. the deployment of AI algorithms and models directly on local edge devices such as mobile phones, are driving the development of smaller, more efficient models that achieve better results on very specific tasks. This opens up opportunities for smaller players such as DeepSeek to compete with larger models and capture valuable use cases.
The build or buy dilemma: what should enterprises do?
For large organisations with proprietary data and complex needs, building custom AI models can be an advantage. However, the costs and complexity of AI development – especially in terms of talent and infrastructure – remain significant barriers.As such, most smaller businesses won’t be able to justify building AI in-house, making AI-as-a-Service (AIaaS) platforms a go-to solution. These pre-built AI solutions offer a cost-effective and scalable way to implement AI.
What’s next for AI and business?
As AI continues to disrupt industries, several trends will shape its future, further enhancing the value of data and infrastructure:
- AI regulation and privacy: With growing concerns about data privacy and increasing regulation, solutions offering high-quality AI in line with data security and compliance will lead the market.
- AI talent: The AI talent gap will drive demand for self-service tools and end-to-end solutions, allowing businesses to deploy AI without large in-house teams.
- Scalability: From data collection to cost efficiency, scale will determine long-term success and profitability, scalable solutions becoming frontrunners in this emerging space.
Conclusion: embracing the AI-first future
The AI revolution is reshaping business models through three core areas: data-related software, infrastructure and AI applications with unique datasets.
In this new reality, data emerges as both an entry ticket and the last defensible moat in the AI space. Infrastructure solutions will help solve AI’s cost challenge, while smaller, specialised AI leveraging unique datasets will emerge to deliver high accuracy and reliability.
With AI inference costs and shifting business models driving businesses towards usage-based pricing, companies need to adapt their business strategies to remain sustainable. Many will find that AIaaS offers the necessary scalability and cost structure. Together, these elements underscore a new era of operational strategies and business models powered by AI.